Stable Video Diffusion: a Free AI Video Generator
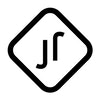
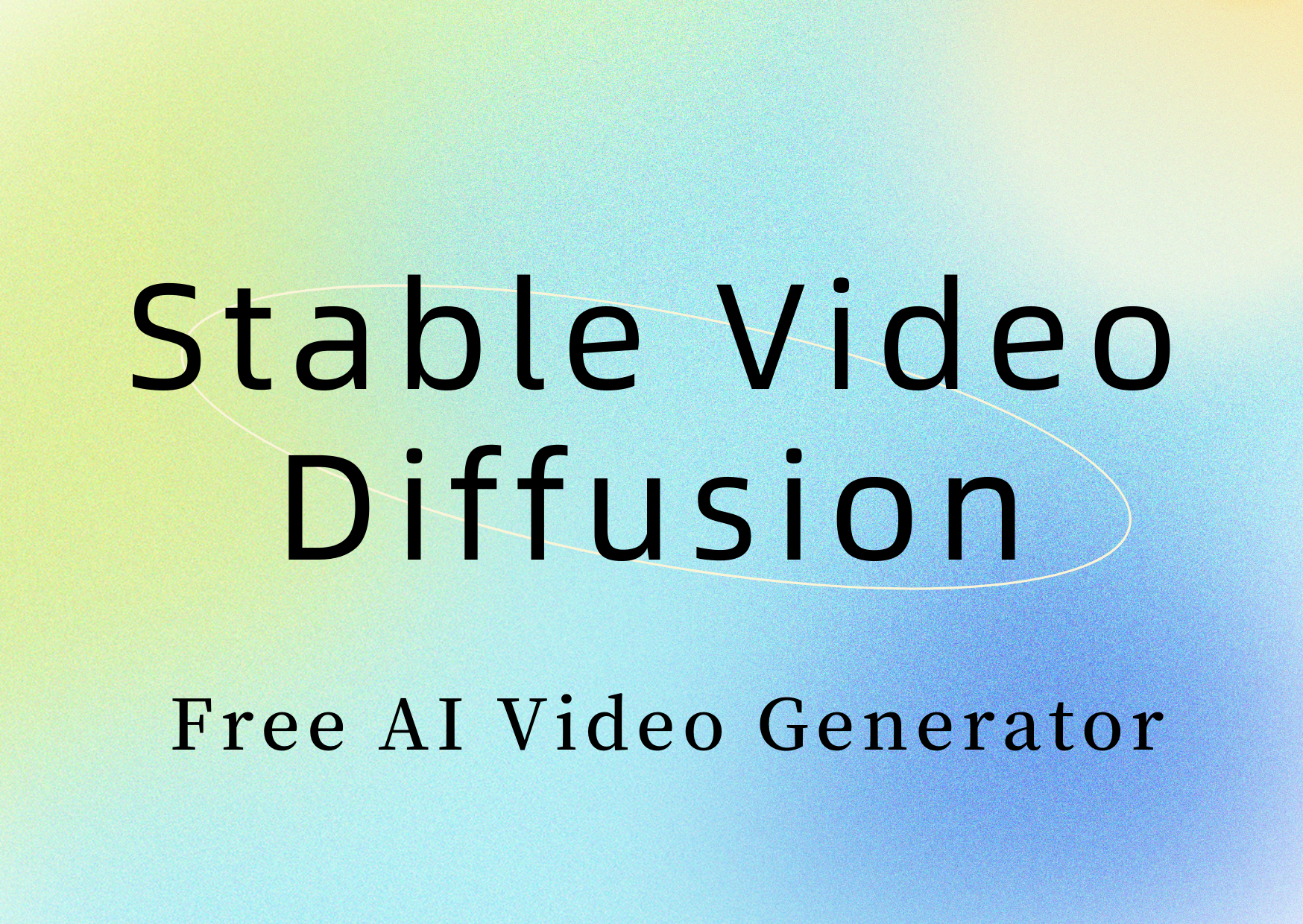
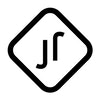
Stability AI, a renowned company in AI graphics, has finally ventured into AI-generated videos. This week, the AI community is abuzz with discussions about the Stable Video Diffusion model, which is based on Stable Diffusion. Many are expressing their excitement, saying, "We've been waiting for this."
What is Stable Video Diffusion?
Stable Video Diffusion, an extension of Stability AI's original Stable Diffusion image generation model, has entered the scene as one of the few open-source or commercially available video generation models. While not yet universally accessible, Stability AI has opened a waitlist for users interested in trying out Stable Video Diffusion. You can submit your waitlist application here.
According to reports, Stable Video Diffusion seamlessly adapts to various downstream tasks, including synthesizing multiple views from a single image through fine-tuning on multi-view datasets. Stability AI plans to build and expand various models around this foundational one, similar to the ecosystem developed around stable diffusion.
It is released in two forms, capable of generating videos at customizable frame rates ranging from 3 to 30 frames per second, resulting in 14 and 25 frames videos.
External evaluations confirm that these models by Stability AI surpass leading closed-source models in user preference studies. However, Stability AI emphasizes that, at this stage, Stable Video Diffusion is not suitable for real-world or direct commercial applications. Subsequent improvements will be made based on user insights and feedback regarding safety and quality.
For a deep dive into the research paper, you can visit here.
Stable Video Diffusion adds to Stability AI's diverse family of open-source models, showcasing their commitment to enhancing AI across various modalities, including images, language, audio, 3D, and code.
The Mechanics of this AI Tool
Stable Video Diffusion, as a high-resolution video latent diffusion model, has achieved state-of-the-art levels in text-to-video or image-to-video synthesis. Recently, the latent diffusion model, originally trained for 2D image synthesis, evolved into a video generation model by introducing a temporal layer and fine-tuning on a small high-quality video dataset. However, training methods in the literature vary significantly, and there is yet to be a consensus on a unified strategy for organizing video data.
In the research paper for Stable Video Diffusion, Stability AI identifies and evaluates three distinct stages for successfully training video latent diffusion models: text-to-image pretraining, video pretraining, and high-quality video fine-tuning. They demonstrate the importance of a carefully curated pretraining dataset for generating high-quality videos and introduce a systematic planning process for training a robust foundational model, including captioning and filtering strategies.
The paper also explores the impact of fine-tuning the base model on high-quality data, leading to a text-to-video model comparable to closed-source video generators. This model provides powerful motion representations for downstream tasks, such as image-to-video generation and adaptability to camera motion-specific LoRA modules. Additionally, the model offers robust multi-view 3D priors, serving as the foundation for multi-view diffusion models. It generates multiple views of an object in a feed-forward manner, requiring minimal computational resources and outperforming image-based methods.
To sum up, successful training of this model involves three stages:
Stage 1: Image Pretraining The paper views image pretraining as the first stage in the training pipeline. It establishes the initial model based on Stable Diffusion 2.1, providing the video model with powerful visual representations. Results indicate a preference for the quality and prompt tracking of the image pretraining model.
Stage 2: Video Pretraining Dataset The authors rely on human preferences as signals to create a suitable pretraining dataset, called LVD (Large Video Dataset), consisting of 580M annotated video clips. The paper emphasizes the importance of a well-prepared pretraining dataset and introduces annotation strategies using dense optical flow and optical character recognition to eliminate clips with excessive text.
Stage 3: High-Quality Fine-Tuning To analyze the impact of video pretraining on the final stage, the paper fine-tunes three models that differ only in their initialization. Results indicate the effectiveness of high-quality fine-tuning.
It looks like a promising start. When can we expect AI to directly generate a full-length movie?
If you're eager to learn more about Stable Video Diffussion, check out some of these articles:
- Google Gemini is Here And Its Better Than GPT-4
- How to Make AI VIDEOS (with Stable Diffusion, Runway)
- Summary of "Stable Video Diffusion - Local Install Guide" Video
- How to run Stable Video Diffusion in ComfyUI ?img2vid
- How to Deploy Stable Video Diffusion in Colab?
- How to install Stable Video Difussion?
- Stable Video Diffusion: a Free AI Video Generator